Lesson 12 - Emerging Technologies
Site: | Technology-Enabled Learning Lounge |
Course: | Teaching in a Digital Age |
Book: | Lesson 12 - Emerging Technologies |
Printed by: | Guest user |
Date: | Friday, 26 April 2024, 3:08 PM |
Table of contents
- 1. Watch this Video on Emerging Technologies
- 2. Emerging Technologies: Serious Games and Gamification
- 3. Emerging Technologies: Virtual and Augmented Reality
- 4. Emerging Technologies: Artificial Intelligence
- 5. Emerging Technologies: Conclusion and Summary
- 6. Activity (Reflective Thinking, Note Taking and Discussion)
- 7. Key Takeaways
1. Watch this Video on Emerging Technologies
2. Emerging Technologies: Serious Games and Gamification
2.1. The Challenge of Emerging Technologies
It is not uncommon for a school principal, a college VP Education, or a university president to go to a conference and come back thrilled about the potential of the latest technology for teaching and learning. They are victims of what the consulting firm Gartner calls the hype cycle.
A new technology triggers excitement, the media picks up on it, the technology reaches a peak of inflated expectations, it starts to get more widely applied, disillusionment sinks in when faced with the realities of implementation, then the technology starts to find its niche as better understanding of its strengths and weaknesses emerge, eventually reaching a plateau of productivity, where it works well within its limits. MOOCs are an excellent example of this, with most knowledgeable observers in 2019 placing them towards the top of the slope of enlightenment or just emerging on to the plateau of productivity (see, for instance, Web Courseworks, 2018).
New technologies that have educational applications are constantly emerging. For instance, in the first edition of this book (written in 2015) there was no extensive discussion of artificial intelligence, virtual reality or serious games, yet four years later they are now at the forefront of many discussions about the future of digital learning, which is why this section has been added. There are several other technologies that could be included, but many of these will be subsumed under artificial intelligence.
I will not be able to go into depth about any of these three technologies (each deserves its own book), but they are significant enough to bring them to your attention. Once again, I will focus on their potential affordances, although it must be recognized that with all emerging technology, it may take time to identify all their advantages and disadvantages.
2.2. Serious Games
Gartner’s hype cycle is best considered as a way of thinking about emerging technologies, rather than as a factual representation of their development. For instance, serious games are more of a slow burner. There have never been vastly inflated expectations about their likely impact on education; indeed, for a long time, they have been written off as too expensive or not appropriate for serious education. However, that view has been changing in recent years.
What are Serious Games?
There are several different definitions of serious games. I have included two definitions that cover both educational and corporate settings.
The Financial Times Lexicon offers the following definition:
Serious games are games designed for a purpose beyond pure entertainment. They use the motivation levers of game design – such as competition, curiosity, collaboration, individual challenge – and game media, including board games through physical representation or video games, through avatars and 3D immersion, to enhance the motivation of participants to engage in complex or boring tasks. Serious games are therefore used in a variety of professional situations such as education, training, assessment, recruitment, knowledge management, innovation and scientific research.
Zhonggen (2019) provides this definition in his comprehensive review of the research on serious games:
Serious games are referred to as entertaining tools with a purpose of education, where players cultivate their knowledge and practice their skills through overcoming numerous hindrances during gaming.
It is important to distinguish between serious games, game-based learning and gamification because of the differences in their purpose, approach and impact on learning.
- Game-based learning refers to “the pedagogical approach of utilizing games in education” (Anastasiadis, Lampropoulos and Siakas, 2018)
- Gamification is defined as the “use of game design elements in non-game contexts” (Deterding et al., 2011)
Note that serious games are not necessarily digital. However, whether digital or not, they are governed by similar principles of design, such as mechanics, dynamics and aesthetics (Hunicke et al., 2004).
Why User Serious Games?
The main reasons offered for using games in education are to:
- Improve students’ motivation to learn
- Engage learners more deeply in the learning process
- Improve learning outcomes
- Improve attendance and participation
However, an extensive review of the literature conducted by Dichev and Dicheva in 2017 found that research remains inconclusive on these assumptions. They also found that:
- The practice of gamifying learning has outpaced researchers’ understanding of its mechanisms and methods;
- Insufficient high-quality evidence exists to support the long-term benefits of serious games in an educational context;
- A limited understanding that how to gamify an activity depends on the specifics of the educational context.
Dichev and Dicheva do conclude though that their study does not mean that gamification cannot be used successfully in a learning context; rather better designs and more research are needed.
Other research tends to be more positive. Hamari et al. (2016) and Clark et al. (2016) found sufficient evidence that, when well designed, and under the right conditions, serious games significantly enhanced student learning relative to nongame conditions.
Zhonggen (2019) found among the ‘ huge number of findings in serious game assisted learning, most …are supportive, coupled with a few negative results.’ However, the main benefits tended to be in the affective domain (student ‘happiness’ and improved social learning and communication) rather than in immediately improved cognitive learning outcomes, except in science (improved retention and holistic understanding), architecture and medicine/health. In the latter, games helped children with autism to learn. Zhonggen reports:
‘Generally, … medical science has recently witnessed clearly more studies on serious game assisted learning compared with other fields and most of studies in medical science supported use of serious games.’
Examples of Serious Games
The Digital Education Strategies team (DES) at Ryerson University has participated in the development of several virtual games simulations including:
Games-based learning: Ryerson University’s Academic Integrity office, in collaboration with DES, developed a digital learning game called Academic Integrity in Space to motivate students to complete self-study training and to learn about the academic integrity, values and behaviours expected of students. The game development team’s objectives were to create a well-designed digital game to meet the learning objectives of making choices, learning by doing, and experiencing situations first-hand, through role-playing.
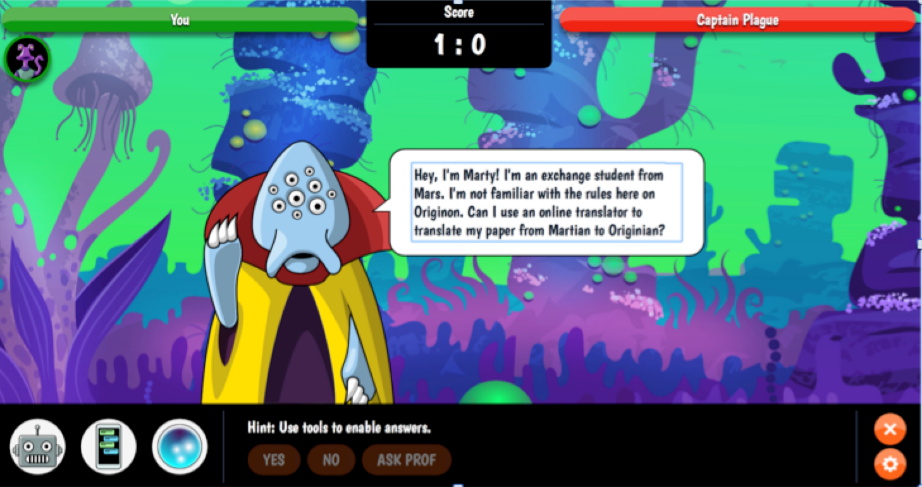
Video Game Simulation: A Home Visit game promotes the application of knowledge and skills related to establishing a therapeutic nurse-client relationship and completing a mental health assessment. Students assume the role of a community health nurse assigned to complete a home visit. Video is used to create an authentic experience, and students have to respond to particularly challenging situations, based on procedures taught elsewhere in the course. Depending on the student response, further video segments are used to provide feedback and to continue to scenarios to test the next appropriate procedure. Professors from Centennial College, Ryerson University and George Brown College are developing a series of open-access video game simulations through a virtual healthcare experience portal.
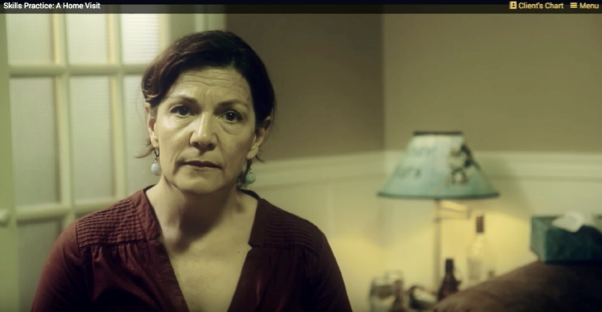
Gamification: Kyle Geske, an instructor at Red River College, Winnipeg, has developed a games-based approach to teaching web design. In his elective course on Full Stack Development of web sites, students have to design a project according to principles provided by the instructor. At each stage of the design process within the project students gain marks, and compete throughout the course with other students, who can see the marks at each stage for all the other students. A student can ‘level up’ their mark by going back and improving on each of the steps of the design. This approach has resulted in an increase in the average end of course grade compared to the more traditional classroom methods. Note this course involves elements of gaming, such as competition, and ‘levelling up’, without using games themselves.
Designing Serious Games
Zhonggen’s review of the literature (2018) highlighted the importance of the following in effective games design:
- Backstory and production
- Realism
- Artificial intelligence and adaptivity
- Interaction
- Feedback and debriefing
- Ease of use
- Surprises
As a result of this prior research, and under the leadership of Naza Djafarova, the Digital Education Strategies team (DES) at the G. Raymond Chang School for Continuing Education at Ryerson University in Toronto developed a practical design guide (2018) for serious game-based learning, based on a games research process. This guide is an open educational resource and is designed to serve three purposes:
- Provide a conceptual framework to guide game design within multidisciplinary teams in higher education
- Offer a methodological guide to running a participatory workshop focused on the pre-production phase of the game development process
- Share resources by making the guide and the design of the workshop available as open educational resources
The games design methodology is an adaptation of the Design, Play, and Experience (DPE) Framework, developed by Winn (2009). The game development process consists of three phases:
- The pre-production phase, during which brainstorming among team members takes place, leading to the design of a paper prototype of the game
- The production phase, when the game is developed; and
- The post-production phase, during which the game is tested and refined before being offered to learners
The Digital Education Strategies team utilized the Design, Play and Experience model to identify four essential educational game elements:
- Learning refers to the content to be learned by players through the game with specific and measurable learning outcomes
- Storytelling refers to the background story of the game and includes a description of the character(s), the setting, and the ultimate goal of the game
- Gameplay refers to the way in which the player interacts with the game, or with other players (if a multiplayer game). It encapsulates the type of activity (e.g., puzzle, trivia, etc.) found in the game
- User Experience refers to the player’s emotions and attitudes while playing the game, as well as how the player interacts with the game
Below figure provides a more detailed representation of the various components of the Ryerson serious game design methodology.
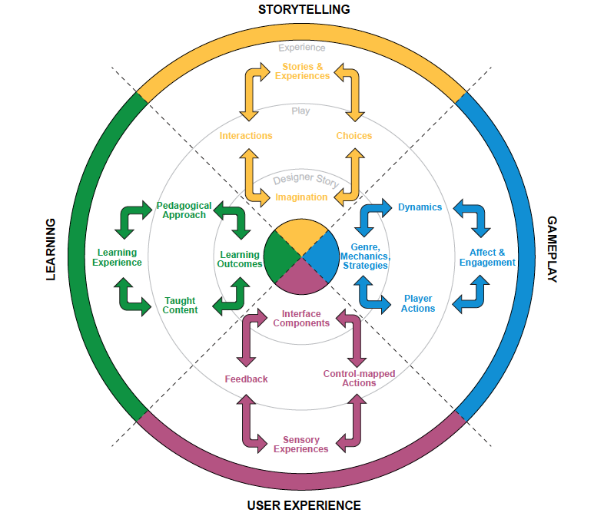
The Digital Education Strategies’ report suggests a workshop approach to serious games design, in which all the key stakeholders (content experts, instructional designers, media producers, and so forth) are involved. Brainstorming in the early stages of design is considered essential. Also built into the design is testing and user feedback before releasing the game.
There are probably other effective design approaches, but the above approach highlights the essential multi-disciplinary approach of serious games design.
Unique Educational Characteristics of Serious Games
These still need to be clearly identified and validated, but two rather different claims are made for serious games:
- The first is that they can increase student motivation and engagement
- The second is that games can be particularly useful for developing the following skills:
- Problem-solving
- Communication skills
- Decision-making
Within specific contexts that approximate to the real world.
2.3. Strengths and Weaknesses
In terms of the hype cycle, serious games are somewhere along the slope of enlightenment. There is not the research yet to move them into the plateau of productivity, but there is enough evidence from practice that they are gaining traction in education.
However, there are a number of reasons why serious games have not become more prevalent in education. The first is philosophical. There is resistance to the idea of games because some see serious games as an oxymoron. How can a game be serious? Many instructors fear that learning could easily be trivialized through games or that games can cover only a very limited part of what learning should be about – it can’t all be fun; that is not the purpose of education. Similarly, many professional game designers are not interested in developing serious games because they fear that if the primary goal is learning and not enjoyment, a focus on education risks killing the main element of a game: being fun to play.
A more pragmatic reason is cost and quality. The assumed high cost of video games has so far acted as a deterrent in education. There is no obvious business plan to justify the investment. The bestselling video games for entertainment for instance cost millions of dollars to produce, on a scale similar to mainstream movies. If games are produced cheaply, won’t the quality – in terms of production standards, narrative/plot, visuals, and learner engagement – suffer, thus making them unattractive for learners?
However, probably the main reason serious games are not more prevalent in education is that most educators simply do not know enough about serious games: what exists, how they can be used, nor how to design them. Experience suggests that there are many possible and realistic applications for serious games in education. There is some evidence (see for instance, Arnab, 2014) that effective serious games can be developed at very little cost.
Nevertheless, there is always a high degree of risk in serious games design. There is no sure way of predicting in advance that a new game will be successful. Some low-cost simple games can work well; some expensively produced games can easily flop. This means careful testing and feedback during development. So serious games should be more seriously considered for teaching in a digital age – but their application needs to be done carefully and professionally.
Thus serious games are relatively high risk, high return activity for teaching in a digital age. Success in serious games means building on best practices in games design, both within and outside education, sharing costs and experience, and collaboration between institutions and games development teams. However, as teaching in a digital age moves more and more towards high-level skills development, experiential learning, and problem-solving in real-world contexts, serious games are bound to play an increasingly important role.
3. Emerging Technologies: Virtual and Augmented Reality
As with serious games, virtual and augmented reality are technologies that have been around for sometimes while making a relatively small impact on education in their earlier development. However, more recent technological developments that have moved virtual worlds from two-dimensions (such as Second Life) into three-dimensional, deeply immersive environments have brought more attention to their potential in education (for a good overview of the history and potential of augmented and virtual reality in education, see Elmqadden, 2019).
3.1. What are Virtual/Augmented/Mixed Reality?
A simple definition of these technologies is ‘human immersion in a synthetic world‘ (Seidel and Chatelier, 1997). The Franklin Institute provides the following more detailed definitions that attempt to distinguish between the different types of ‘synthetic’ worlds:
- Augmented reality (AR) adds digital elements to a live view often by using the camera on a smartphone. Examples of augmented reality experiences include Snapchat lenses and the game Pokémon Go.
- Virtual reality (VR) implies a complete immersion experience that shuts out the physical world. Using VR devices such as HTC Vive, Oculus Rift or Google Cardboard, users can be transported into a number of real-world and imagined environments such as the middle of a squawking penguin colony or even the back of a dragon.
- In a mixed reality (MR) experience, which combines elements of both AR and VR, real-world and digital objects interact. Mixed reality technology is just now starting to take off, with Microsoft’s HoloLens one of the most notable early mixed reality apparatuses.
I will use the term ‘immersive technologies’ for all these technologies. However, verbal descriptions will always be somewhat inadequate in describing what are essentially multi-sensory experiences, combining vision, hearing, and movement. These technologies are something that needs to be experienced rather than explained if they are to be better understood.
3.2. Why Use Immersive Technologies?
There are several reasons why these technologies are beginning to be used more in education:
- The recent development of relatively low cost and easily wearable end-user technology (headsets in particular)
- Deep immersion into three-dimensional, highly realistic learning environments that are strongly compelling/motivating for the end-user
- The ability for end-users to manipulate objects within the three-dimensional environment
- More powerful cloud computing technology that allows for the development of more complex and more realistic learning environments, combined with more advanced developments in mobile technologies and high-speed wireless networks
- The potential for developing a range of skills and knowledge that would be difficult, impossible or dangerous in real-world environments
3.3. Examples of Immersive Environments In Education
Looking at the challenges above, it may be wondered why anyone would bother with immersive technologies in education. However, the potential benefits have barely been explored. I provide examples here that demonstrate both the potential benefits and how some immersive environments can be developed relatively easily. (For a more systematic review of VR applications in higher or post-secondary education, see Radianti et al., 2020)
Virtual Reality
In the Department of Chemistry at the University of Bristol in England, Dr. David Glowacki and his team in their VR laboratory created an interactive molecular dynamics modelling tool in the form of Nano Simbox VR, which allowed anyone to visit and play within the invisible molecular world (O’Connor et al., 2018). The main aim of this particular project was to provide an intuitive feeling of the way molecules operate in multiple dimensions to enable researchers and students to have a better understanding of how nano worlds operate, leading to better hypotheses for testing within this particular domain.
As the authors state in the article:
From a modeling perspective, the nanoscale represents an interesting domain, because the objects of study (for example, molecules) are invisible to the naked eye, and their behavior is governed by physical forces and interactions significantly different from those forces and interactions that we encounter during our day-to-day phenomenological experience. In domains like this, which are imperceptible to the naked eye, effective models are vital to provide the insight required to make research progress…. molecular systems typically have thousands of degrees of freedom. As a result, their motion is characterized by a complicated, highly correlated, and elegant many-body dynamical choreography, which is nonintuitive compared to the more familiar mechanics of objects that we encounter in the everyday physical world. Their combined complexity, unfamiliarity, and importance make molecules particularly interesting candidates for investigating the potential of new digital modeling paradigms.
Glowacki and his team in Science Advances (O’Connor et al., 2018) describe how the VR app enabled researchers to:
- Easily “grab” individual C60 atoms and manipulate their real-time dynamics to pass the C60 back and forth between each other
- Take hold of a fully solvated benzylpenicillin ligand and interactively guide it to dock it within the active site of the TEM-1 β-lactamase enzyme (with both molecules fully flexible and dynamic) and generate the correct binding mode (33), a process that is important to understanding antimicrobial resistance
- Guide a methane molecule (CH4) through a carbon nanotube, changing the screw sense of an organic helicene molecule
- Tie a knot in a small polypeptide [17-alanine (17-ALA)
Building dynamic models that operate not only in real-time but also in three dimensions can require not only specialized virtual reality equipment but more importantly massive amounts of computing power to handle the visual representation and modeling of highly complex, interactive dynamic molecular processes. However, through the use of cloud computing and faster networks, building such models has now become a reality, enabling not only such models to be represented but allowing some degree of real-time manipulation by researchers in different locations but within the same time-frame. The main advantage of the use of a cloud platform is to allow the scaling up of modeling from simple to much more complex dynamic nano interactions and the synchronous sharing of the virtual reality experience with multiple users.
Not all applications of VR though need massive computing power. Other exploratory uses of virtual reality are
- For students to find their way round a complex campus
- In architecture/space planning, allowing clients to understand in three dimensions the final ‘look’ of a building design by virtually walking through it (Brandaõ et al., 2018), Google Blocks, a free software program for developing 3D models, is one tool that can support this kind of application.
- In music: at the University of British Columbia, Dr. Jonathon Girard is exploring the use of VR for learning how to conduct an orchestra (the virtual orchestra ‘responds’ to the hand gestures of the conductor)
- In medicine and health: researchers at UBC are exploring the use of VR for pain management
Augmented Reality
Augmented reality is a simpler immersive technology than virtual reality, often based on apps for mobile phones. For instance, students in the University of British Columbia’s APBI 200 Introduction to Soil Science learn about the effects of topography on the formation of different soil types. The department has developed the Soil TopARgraphy app, which allows viewing and manipulating a terrain model in the Kamloops region of British Columbia. Students learn how topography impacts the distribution of soil orders through its effects on microclimate (i.e. temperature and water). Students are able to view the terrain model with a color-coded elevation map or a satellite image on their mobile phones. Furthermore, students can tap on flags to read about different soil orders, view images, and take a self-study quiz to reinforce their understanding.
For this project, UBC’s Emerging Media Lab built two mobile apps, an AR viewer for students (Android and iOS) and an editor for the instructor (Android). The AR viewer is the app described above to view a predefined terrain. The instructor can customize content with the supplementary editor app. They can update soil location on terrain, description, image, and quizzes.
Other examples of AR applications from UBC:
Dr. Patrick Walls is developing a mobile phone-based app that helps students visualise multivariable functions, in order to learn the underlying concepts at a deeper level much more quickly.
In GEOG 498: Geographies in the Middle East, students learn about the history of the Syrian Civil War and its ongoing developments. The instructor, Dr. Siobhán McPhee, has developed a mobile app that follows the stories of five Syrian refugees who eventually reached Vancouver. Students are forced to make choices (or given a lack of choice), wait, and run/walk with the app to be able to progress the narrative of the experience. The purpose of this project is to evoke empathy and help students understand the emotional consequences of the Syrian Civil War. This app also applies some gamification principles as well.
3.4. Designing Immersive Educational Environments
This technology is so recent that there are few or no accepted best practices developed yet for educational use. Most educational applications to date have been deliberately exploratory in nature. However, there are several stages of development required that will apply to all educational applications of these technologies:
- Identify start-up costs and possible sources of funding: this is not likely to be a cheap exercise, at least initially; for this reason, several universities, such as the University of British Columbia, and Drexel University, have set up their own emerging technologies research labs to experiment with educational applications;
- Define learning outcomes/objectives: what is the learner expected to learn? In the early stages of development, this may be both a brainstorming exercise (preferably including students/end-users) and an iterative process, because the full potential of the technology is not always clear in the first applications. In particular, the instructor needs to have a clear vision of what might be possible using an immersive technology. Thus some familiarity with the technology is essential before starting design;
- Determine where the use of this technology fits within the overall design of a course/program: in other words, what knowledge and skills will be developed within the immersive environment, and how does this integrate with what is being taught in the rest of the course/program?
- Decide between using an existing immersive design/learning environment that can be applied or adapted relatively easily for ‘local’ use; or designing a new immersive environment from scratch. The latter is obviously more expensive and time-consuming and will require a high level of expertise; as a result the pay-off from design from scratch (improved learning outcomes/return on investment) needs to be worth the effort;
- Choice of appropriate/affordable technology. Headsets or mobile apps are the least expensive part of the use of immersive technologies. The main cost will be in developing or adapting the ‘augmented’ or ‘virtual’ world. However, as with serious games, there can be an intermediary step, where an existing ‘world’ can be licensed and adapted for local use (see, for instance, lightwave). In some cases, open access immersive worlds are available for use or adaptation, although they are not common (see opensimulator, art of illusion, or mayaverse, for examples.). Often students can be used to help with programming and design of the environment, as part of their studies, but they will need direction as well as the opportunity to offer creative ideas. Truly interactive virtual worlds where learners/users make decisions and the consequences are ‘programmed’ into the learning environment may require large amounts of computing capacity, such as cloud computing;
· To be effective, the VR environment has to be as authentic or realistic as possible. This means paying as much attention to creating the specific learning context. It will be necessary to decide what parts of the learning will best be done outside the VR/AR experience, and which inside. For instance, the procedures for monitoring the state of a nuclear reactor, for identifying critical incidents, for deciding whether or not or when to shut down the reactor, and for actually shutting down the reactor must also be built into the learning process. Most of this may be taught outside the VR context, but VR can be used to test or develop the skills of applying this knowledge in a realistic, challenging context. In other words, the VR experience needs to be embedded within a broader learning context or environment;
- Testing and adaptation: Design, at least initially, needs to be an iterative process, where ideas are developed and tried, and feedback received and incorporated into the design;
- Assessment: This can be a particular challenge, particularly if new learning outcomes result from the experience. How can assessment best capture what students have learned? Will assessment take place within the ‘virtual’ world, in the real world, or in some other way (and if so, how authentic will such an assessment be)?
- In what ways could the new immersive environment be scaled up to enable costs to be recovered?
- Evaluation: What is the best way to evaluate the success or limitations of the design and application of the immersive world? How best to disseminate the knowledge and experience gained?
These may appear formidable challenges, but the potential benefits could be considerable.
The development of fully immersive technologies is so recent that it is premature to try to identify all the educational affordances that are unique to this medium. New applications are being explored all the time. Most of the evidence is qualitative, based on people’s personal experience of using the technology. Empirical evidence that validates specific educational affordances of VR/AR in terms of improved learning outcomes is currently lacking. However, the potential of VR/AR in terms of assisting learning can be identified.
First of all, many of the affordances or educational characteristics of other media, and in particular video, will apply to VR and AR, but often more intensely, because of the immersive experience.
Virtual and augmented reality applications can provide students with a deep, intuitive understanding of phenomena that are otherwise difficult if not impossible to achieve in other ways. This enables students who often struggle with the abstract nature of an academic subject to understand in more concrete terms what the abstractions mean or represent. This intuitive understanding is critical not only for deeper understanding but also for breakthroughs in research and applications of science.
Educational applications where the cost of alternative or traditional ways of learning are too expensive or too dangerous will be particularly suitable for virtual reality applications. Examples might be emergency management, such as shutting down an out-of-control nuclear reactor, or defusing a bomb, or managing a fire on an oil tanker, or exploring inside the physical structure of a human brain. In particular, VR would be appropriate for learning in contexts where real environments are not easily accessible, or where learners need to cope with strong emotions when making decisions or operating under pressure in real-time.
AR, which is often easier to design and implement, enables learners to practice applications of knowledge in semi-realistic contexts.
However, at the time of writing, we are just beginning to understand the potential of this medium. Over time, the educational affordances of this medium will become much clearer.
3.5. Strengths and Weaknesses
VR is not just a fad that will disappear. There are already a large number of commercial applications, mainly in entertainment and public relations, but also increasingly for specific areas of education and training. There is already a lot of excellent, off-the-shelf software for creating VR environments, and the cost of hardware is dropping rapidly (although good quality headsets and other equipment are still probably too expensive for required use by large numbers of students).
The fields of application of this technology are unlimited: training in the use of complex equipment, simulation of surgical procedures, architectural design testing, the reconstruction of sites in archeology, virtual museum visits, treatment of pain and phobias, and many other possibilities.
To enable the more emotional aspects of decision making to be handled, the immersive experience needs to be realistic. This will probably require high-quality media production. Thus VR may often need to be combined with simulation design, quality media production, and powerful computing to be educationally effective, again pushing up the cost. For these reasons, medicine is a particularly likely area for development, where traditional training costs are really high or where training is difficult to provide with real patients.
Once again, though, applications will tend to be very specific to the needs of a particular subject area. This means designers must include subject specialists with a deep understanding of the field who can combine the power of the technology with the needs of learners in a particular learning context. VR in particular requires instructors with imagination and creativity, working with other professionals such as media producers, learners themselves, as well as specialists in VR design.
What has inhibited widespread educational use of earlier two-dimensional VR developments such as Second Life has been the high cost and difficulty of creating the graphics and contexts for learning. Thus even if the hardware and software costs for VR are low enough for individual student use, the high production costs of creating realistic educational contexts and scenarios are likely to inhibit its general use.
Some caution is also needed in assuming that people will behave the same in real life as they do in VR environments. Gallup et al. (2019) found a major difference in the influence of social factors within real-world and virtual environments: social cues in actual reality appear to dominate and supersede those in VR. One of the authors, Alan Kingstone, concluded:
“Using VR to examine how people think and behave in real life may very well lead to conclusions that are fundamentally wrong. This has profound implications for people who hope to use VR to make accurate projections regarding future behaviors. For example, predicting how pedestrians will behave when walking amongst driverless cars, or the decisions that pilots will make in an emergency situation. Experiences in VR may be a poor proxy for real life.”
Rolfsen, 2019
This means we need more experimentation. This is still a relatively new technology, and there may be very simple ways to use it in education that are not costly and meet needs that cannot be easily met in traditional teaching or with other existing technology. For this to happen, though, educators, software developers, and media producers need to come together to play, experiment, test, and evaluate.
Nevertheless, VR and AR are exciting technologies with the potential to change radically conventional learning processes.
4. Emerging Technologies: Artificial Intelligence
4.1. Focusing on AI’s Affordances for Teaching and Learning
Artificial intelligence (AI) is a daunting topic as there are so many issues with respect to its use in education. AI is also currently going through yet another period of extreme hype as a panacea for education, currently being at the top of the peak of inflated expectations, but this hype is driven mainly by successful applications outside the field of education, such as in finance and marketing. Furthermore, the term ‘AI’ is increasingly being used (incorrectly) as a general term for any computational activity.
Even in education, there are very different possible areas of application of AI. Zeide (2019) makes a very useful distinction between institutional, student support, and instructional applications (See Figure below).
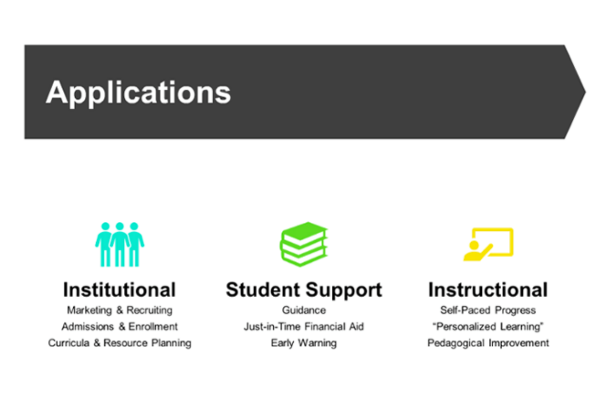
Although AI applications for institutional or student support purposes are very important, this chapter is focused on the pedagogical affordances of different media and technologies (what Zeide calls ‘instructional’ applications). In particular, the focus in this section will be on the role of AI as a form of media or technology for teaching and learning, its pedagogical affordances, and its strengths and weaknesses in this area.
Moreover, AI is really a sub-set of computing. Thus, all the general affordances of computing in education set out in this chapter will apply to AI. This section aims to tease out the extra potential that AI can offer in teaching and learning. This will mean particularly focusing on its role as a medium rather than a general technology in teaching, which means looking at a wider context than just the computational aspects of AI, in particular its pedagogical role.
4.2. What is Artificial Intelligence?
The original definition of artificial intelligence by McCarthy (1956, cited in Russell & Norvig, 2010) is:
Every aspect of learning or any other feature of intelligence can in principle be so precisely described that a machine can be made to simulate it. An attempt will be made to find how to make machines use language, form abstractions and concepts, solve kinds of problems now reserved for humans, and improve themselves.
Zawacki-Richter et al. (2019), in a review of the literature on AI in higher education, report that those authors that defined artificial intelligence tended to describe it as:
Intelligent computer systems or intelligent agents with human features, such as the ability to memorize knowledge, to perceive and manipulate their environment in a similar way as humans, and to understand human natural language.
Klutka et al. (2018) also, define AI in terms of what it can do in higher education (Figure 21 below)
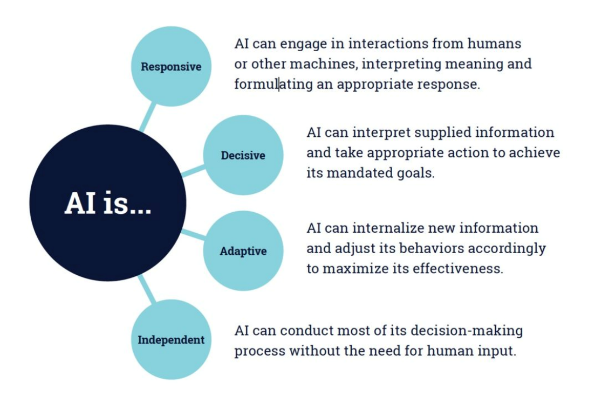
There are three basic computing requirements that set ‘modern’ AI apart from other computing applications:
- Access to massive amounts of data
- Computational power on a large scale to manage and analyze the data
- Powerful and relevant algorithms for the data analysis
4.3. Why Use Artificial Intelligence for Teaching and Learning?
There are two somewhat different goals for the general use of artificial intelligence. The first is to increase the efficiency of a system or organization, primarily by reducing the high costs of labour, namely by replacing relatively expensive human workers with relatively less costly machines (automation). In education in particular, the main cost is that of teachers and instructors. Politicians, entrepreneurs and policy makers increasingly see a move to automation as a way of reducing the costs of education.
The second is to increase the effectiveness of teaching and learning, in economic terms to increase outputs: better learning outcomes and greater benefits for the same or more cost. With this goal, AI would be used alongside or supporting teachers and instructors.
Klutka et al. (2018) provide a general statement of the potential of AI in higher education ‘instruction’ through Figure below.
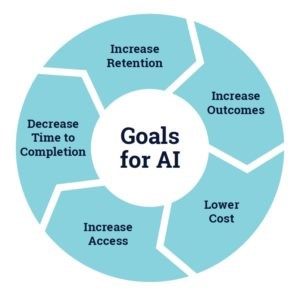
These are understandable goals, but we shall see later in this section that such goals to the date is mainly aspirational rather than real.
In terms of this book, a key focus is on developing the knowledge and skills required by learners in a digital age. The key test then for artificial intelligence is to what extent it can assist in the development of these higher-level skills.
4.4. Affordances and Examples of AI Use in Teaching and Learning
Zawacki-Richter et al. (2019) in a review of the literature on AI in education initially identified 2,656 research papers in English or Spanish, then narrowed the list down by eliminating duplicates, limiting publication to articles in peer-reviewed journals published between 2007 and 2018, and eliminating articles that turned out in the end not to be about the use of AI in education. This resulted in a final 145 articles which were then analyzed. Zawacki-Richter et al. then classified these 145 papers into different uses of AI in education. This section draws heavily on this classification. (It should be noted that within the 145 articles, only 92 were focused on instruction/student support. The rest were on institutional uses such as identifying at risk students before admission).
The Zawacki-Richter study offers one insight into the main ways that AI has been used in education for teaching and learning over the ten years between 2007 and 2018, the closest we can come to ‘affordances’. First, three main general ‘instructional’ categories (with considerable overlap) from the study are provided below, followed by some specific examples. (I have omitted Zawacki-Richter et al.’s category of profiling and prediction concerned with administrative issues such as admissions, course scheduling, and early warning systems for students at risk.)
Intelligent Tutoring Systems (29 out of 92 Articles Reviewed by Zawacki-Richter Et Al.)
Intelligent tutoring systems:
- Provide teaching content to students and, at the same time, support them by giving adaptive feedback and hints to solve questions related to the content, as well as detecting students’ difficulties/errors when working with the content or the exercises.
- Curate learning materials based on student needs, such as by providing specific recommendations regarding the type of reading material and exercises done, as well as personalized courses of action.
- Facilitate collaboration between learners, for instance, by providing automated feedback, generating automatic questions for discussion, and the analysis of the process.
Assessment and Evaluation (36 out of 92)
AI supports assessment and evaluation through:
- Automated grading
- Feedback, including a range of student-facing tools, such as intelligent agents that provide students with prompts or guidance when they are confused or stalled in their work
- Evaluation of student understanding, engagement and academic integrity
Adaptive Systems and Personalization (27 out of 92)
AI enables adaptive systems and the personalization of learning by:
- Teaching course content then diagnosing strengths or gaps in student knowledge, and providing automated feedback;
- Recommending personalized content;
- Supporting teachers in learning design by recommending appropriate teaching strategies based on student performance;
- Supporting representation of knowledge in concept maps.
Klutka et al. (2018) identified several uses of AI for teaching and learning in universities in the USA. ECoach, developed at the University of Michigan, provides formative feedback for a variety of mainly large classes in the STEM field. It tracks students progress through a course and directs them to appropriate actions and activities on a personalized basis. Other applications listed in the report include sentiment analysis (using students’ facial expressions to measure their level of engagement in studying), an application to monitor student engagement in discussion forums, and organizing commonly shared mistakes in exams into groups for the instructor to respond once to the group rather than individually.
Chatbots
A chatbot is programming that simulates the conversation or ‘chatter’ of a human being through text or voice interactions (Rouse, 2018). Chatbots in particular is a tool used to automate communications with students. Bayne (2014) describes one such application in a MOOC with 90,000 subscribers. Much of the student activity took place outside the Coursera platform within social media. The five academics teaching the MOOC were all active on Twitter, each with large networks, and Twitter activity around the MOOC hashtag (#edcmooc) was high across all instances of the course (for example, a total of around 180,000 tweets were exchanged on the first offering of the MOOC). A ‘Teacherbot’ was designed to roam the tweets using the course Twitter hashtag, using keywords to identify ‘issues’ then choosing pre-designed responses to these issues, which often entailed directing students to more specific research on a topic. For a review of research on chatbots in education, see Winkler and Söllner (2018).
Automated Essay Grading
Natural language processing (NLP) artificial intelligence systems – often called automated essay scoring engines – are now either the primary or secondary grader on standardized tests in at least 21 states in the USA (Feathers, 2019). According to Feathers:
Essay-scoring engines don’t actually analyze the quality of writing. They’re trained on sets of hundreds of example essays to recognize patterns that correlate with higher or lower human-assigned grades. They then predict what score a human would assign an essay, based on those patterns.
Feathers though claims that research from psychometricians and AI experts show that these tools are susceptible to a common flaw in AI: bias against certain demographic groups (see Ongweso, 2019).
Lazendic et al. (2018) offer a detailed account of the plan for machine grading in Australian high schools. They state:
It is …crucially important to acknowledge that the human scoring models, which are developed for each NAPLAN writing prompt, and their consistent application, ensure and maintain the validity of NAPLAN writing assessments. Consequently, the statistical reliability of human scoring outcomes is fundamentally related to and is the key evidence for the validity of NAPLAN writing marking.
In other words, the marking must be based on consistent human criteria. However, it was announced later (Hendry, 2018) that Australian education ministers agreed not to introduce automated essay marking for NAPLAN writing tests, heeding calls from teachers’ groups to reject the proposal.
Perelman (2013) developed a computer program called the BABEL generator that patched together strings of sophisticated words and sentences into meaningless gibberish essays. The nonsense essays consistently received high, sometimes perfect, scores when run through several different scoring engines. See also Mayfield, 2013, and Parachuri, 2013, for thoughtful analyses of the issues in the automated marking of writing.
At the time of writing, despite considerable pressure to use automated essay grading for standardized exams, the technology still has many questions lingering over it.
4.5. Strengths and Weaknesses
There are several ways to assess the value of the teaching and learning affordances of particular applications of AI in teaching and learning:
- Is the application based on the three core features of ‘modern’ AI: massive data sets, massive computing power; powerful and relevant algorithms?
- Does the application have clear benefits in terms of affordances over other media, and particularly general computing applications?
- Does the application facilitate the development of the skills and knowledge needed in a digital age?
- Is there unintended bias built into the algorithms? Does it appear to discriminate against certain categories of people?
- Is the application ethical in terms of student and teacher/instructor privacy and their rights in an open and democratic society?
- Are the results of the application ‘explainable’? For example, can a teacher or instructor or those responsible for the application understand and explain to students how the results or decisions made by the AI application were reached?
These issues are addressed below.
Is it Really a 'Modern' AI Application in Teaching and Learning?
Looking at the Zawacki-Richter et al. study and many other research papers published in peer-reviewed journals, very few so-called AI applications in teaching and learning meet the criteria of massive data, massive computing power and powerful and relevant algorithms. Much of the intelligent tutoring within conventional education is what might be termed ‘old’ AI: there is not a lot of processing going on, and the data points are relatively small. Many so-called AI papers focused on intelligent tutoring and adaptive learning are really just general computing applications.
Indeed, so-called intelligent tutoring systems, automated multiple-choice test marking, and automated feedback on such tests have been around since the early 1980s. The closest to modern AI applications appear to be automated essay grading of standardized tests administered across an entire education system. However, there are major problems with the latter. More development is clearly needed to make automated essay grading a valid exercise.
The main advantage that Klutka et al. (2018) identify for AI is that it opens up the possibility for higher education services to become scalable at an unprecedented rate, both inside and outside the classroom. However, it is difficult to see how ‘modern’ AI could be used within the current education system, where class sizes or even whole academic departments, and hence data points, are relatively small, in terms of the numbers needed for ‘modern’ AI. It cannot be said to date that modern AI has been tried, and failed, in teaching and learning; it’s not really even been tried.
Applications outside the current formal system are more realistic, for MOOCs, for instance, or for corporate training on an international scale, or for distance teaching universities with very large numbers of students. The requirement for massive data does suggest that the whole education system could be massively disrupted if the necessary scale could be reached by offering modern AI-based education outside the existing education systems, for instance by large Internet corporations that could tap their massive markets of consumers.
However, there is still a long way to go before AI makes that feasible. This is not to say that there could not be such applications of modern AI in the future, but at the moment, in the words of the old English bobby, ‘Move along, now, there’s nothing to see here.’
However, for the sake of argument, let’s assume that the definition of AI offered here is too strict and that most of the applications discussed in this section are examples of AI. How do these applications of AI meet the other criteria above?
Do the Applications Facilitate the Development of the Skills and Knowledge Needed in a Digital Age?
This does not seem to be the case in most so-called AI applications for teaching and learning today. They are heavily focused on content presentation and testing for understanding and comprehension. In particular, Zawacki-Richter et al. make the point that most AI developments for teaching and learning – or at least the research papers – are by computer scientists, not educators. Since AI tends to be developed by computer scientists, they tend to use models of learning based on how computers or computer networks work (since of course, it will be a computer that has to operate the AI). As a result, such AI applications tend to adopt a very behaviorist model of learning: present/test/feedback. Lynch (2017) argues that:
If AI is going to benefit education, it will require strengthening the connection between AI developers and experts in the learning sciences. Otherwise, AI will simply ‘discover’ new ways to teach poorly and perpetuate erroneous ideas about teaching and learning.
Comprehension and understanding is indeed important foundational skills, but AI so far is not helping with the development of higher-order skills in learners of critical thinking, problem-solving, creativity and knowledge-management. Indeed, Klutka et al. (2018) claim that that AI can handle many of the routine functions currently done by instructors and administrators, freeing them up to solve more complex problems and connect with students on deeper levels. This reinforces the view that the role of the instructor or teacher needs to move away from the content presentation, content management, and testing of content comprehension – all of which can be done by computing – towards skills development. The good news is that AI used in this way supports teachers and instructors but does not replace them. The bad news is that many teachers and instructors will need to change the way they teach, or they will become redundant.
Is There Unintended Bias in the Algorithms?
It could be argued that all AI does is to encapsulate the existing biases in the system. The problem though is that this bias is often hard to detect in any specific algorithm, and that AI tends to scale up or magnify such biases. These are issues more for institutional uses of AI, but machine-based bias can discriminate against students also in a teaching and learning context as well, and especially in the automated assessment.
Is the Application Ethical?
There are many potential ethical issues arising from the use of AI in teaching and learning, mainly due to the lack of transparency in the AI software, and particularly the assumptions embedded in the algorithms. The literature review by Zawacki-Richter et al. (2019) concluded:
…A stunning result of this review is the dramatic lack of critical reflection of the pedagogical and ethical implications as well as risks of implementing AI applications in higher education.
What data are being collected, who owns or controls it, how is it being interpreted, how will it be used? Policies will need to be put in place to protect students and teachers/instructors (see for instance the U.S. Department of Education’s student data policies for schools), and students and teachers/instructors need to be involved in such policy development.
Are the Results Explainable?
The biggest problem with AI generally, and in teaching and learning in particular, is the lack of transparency. How did it give me this grade? Why I am directed to this reading rather than that one? Why isn’t my answer acceptable? Lynch (2017) argues that most data collected about student learning is indirect, inauthentic, lacking demonstrable reliability or validity, and reflecting unrealistic time horizons to demonstrate learning.
‘Current examples of AIEd often rely on …. poor proxies for learning, using data that is easily collectable rather than educationally meaningful.’
4.6. Some Perspectives
Dream On, AI Enthusiasts
In terms of what AI is actually doing now for teaching and learning, the dream is way beyond the reality. What works well in finance or marketing or astronomy does not necessarily translate to teaching and learning contexts. In doing the research for this section, it proved very difficult to find any compelling examples of AI for teaching and learning, compared with serious games or virtual reality. It is always hard to prove a negative, but the results to date of applying AI to teaching and learning are extremely limited and disappointing.
This is mainly due to the difficulty of applying ‘modern’ AI at scale in a very fragmented system that relies heavily on relatively small class sizes, programs, and institutions. Probably for modern AI to ‘work’, a totally different organizational structure for teaching and learning would be needed. But be careful what you wish for.
There is a strong affective or emotional influence in learning. Students often learn better when they feel that the instructor or teacher cares. In particular, students want to be treated as individuals, with their own interests, ways of learning, and some sense of control over their learning. Although at a mass level human behaviour is predictable and to some extent controllable, each student is an individual and will respond slightly differently from other students in the same context. Because of these emotional and personal aspects of learning, students need to relate in some way to their teacher or instructor. Learning is a complex activity where only a relatively minor part of the process can be effectively automated. Learning is an intensely human activity, that benefits enormously from personal relationships and social interaction. This relational aspect of learning can be handled equally well online as face-to-face, but it means using computing to support communication as well as delivering and testing content acquisition.
Not Fit for Purpose
Above all, AI has not progressed to the point yet where it can support the higher levels of learning required in a digital age or the teaching methods needed to do this, although other forms of computing or technology, such as simulations, games and virtual reality, can.
In particular, AI developers have been largely unaware that learning is developmental and constructed, and instead have imposed an old and less appropriate method of teaching based on behaviorism and an objectivist epistemology. However, to develop the skills and knowledge needed in a digital age, a more constructivist approach to learning is needed. There has been no evidence to date that AI can support such an approach to teaching, although it may be possible.
AI's Real Agenda
AI advocates often argue that they are not trying to replace teachers but to make their life easier or more efficient. This should be taken with a pinch of salt. The key driver of AI applications is cost reduction, which means reducing the number of teachers, as this is the main cost in education. In contrast, the key lesson from all AI developments is that we will need to pay increased attention to the affective and emotional aspects of life in a robotic-heavy society, so teachers will become even more important.
Another problem with artificial intelligence is that the same old hype keeps going round and round. The same arguments for using artificial intelligence in education go back to the 1980s. Millions of dollars went into AI research at the time, including into educational applications, with absolutely no payoff.
There have been some significant developments in AI since then, in particular pattern recognition, access to and analysis of big data sets, powerful algorithms, leading to formalized decision-making within limited boundaries. The trick though is to recognize exactly what kind of applications these new AI developments are good for, and what they cannot do well. In other words, the context in which AI is used matters, and needs to be taken account of. Teaching and learning is a particularly difficult environment then for AI applications.
Defining AI's Role in Teaching and Learning
Nevertheless, there is plenty of scope for useful applications of AI in education, but only if there is continuing dialogue between AI developers and educators as new developments in AI become available. But that will require being very clear about the purpose of AI applications in education and being wide awake to the unintended consequences.
In education, AI is still a sleeping giant. ‘Breakthrough’ applications of AI for teaching and learning are probably not going to come from the mainstream universities and colleges, but from outside the formal post-secondary system, through organizations such as LinkedIn, lynda.com, Amazon or Coursera, that have access to large data sets that make the applications of AI scalable and worthwhile (to them). However, this would pose an existential threat to public schools, colleges and universities. The issue then becomes: what system is best to protect and sustain the individual in a digital age: multinational corporations using AI for teaching and learning; or a public education system with human teachers using AI as support for learners?
The key question then is whether technology should aim to replace teachers and instructors through automation, or whether technology should be used to empower not only teachers but also learners. Above all, who should control AI in education: educators, students, computer scientists, or large corporations? These are indeed existential questions if AI does become immensely successful in reducing the costs of teaching and learning: but at what cost to us as humans? Fortunately, AI is not yet in a position to provide such a threat, but it may well do so soon.
5. Emerging Technologies: Conclusion and Summary
5.1. Comparing the Three Emerging Technologies
This section has looked at three very different emerging technologies: serious games; immersive technologies; and artificial intelligence. Each has the potential to influence profoundly teaching and learning in a digital age.
Both serious games and immersive technologies such as virtual and augmented reality will be extremely valuable in ‘niche’ areas of teaching and learning. They both have the potential to develop some of the higher-order learning skills of problem solving, analysis, intuitive thinking, and creative thinking, and also can be used to develop affective skills, such as empathy.
However, neither is likely to be a ‘core’ technology that will be extensively used across all forms of teaching. Also, both need significant investment of time and possibly money if they are to be of good quality for teaching purposes. In particular, they will need a multi-disciplinary team approach to design and development.
Therefore, it will be essential to choose the right kind of project, such as topics that are difficult to teach using other methods, or projects aimed at learners who struggle with more conventional teaching methods. Above all, it will be necessary to identify and exploit the optimum educational affordances of these two technologies.
Artificial intelligence is somewhat different to the other two emerging technologies. Artificial intelligence to date manages well the presentation and testing of content acquisition, comprehension, and understanding, but so far has not shown much promise in supporting the development or assessment of the higher-level cognitive skills needed in a digital age. However, by focusing on supporting learners’ comprehension and understanding, AI can free up human teachers and instructors to focus their time on the development of these higher-order skills. Again, this emphasizes the importance of teachers and instructors moving their focus away from content delivery – which AI can increasingly manage well – and focusing more on teaching methods that support higher-order skills development.
Furthermore, these three technologies are not really separate and unrelated but will become increasingly integrated. AI applications could improve the power and range of both serious games and virtual reality. Games can be designed within a virtual reality. The extent to which these technologies become feasible in education will depend heavily on applications outside education which can then be carried over and adapted for educational purposes.
Again, though we come back to three critical issues:
- What are the educational goals of the application?
- To what extent does the application help with the development of higher-order cognitive and/or affective skills?
- What are the costs and organizational implications of such applications within education?
5.2. Lessons to be Learned from the Use of Emerging Technologies
New technology developments show no sign of slowing down. Over time, other new technologies will emerge beside the three technologies discussed in this section. Educators will continue to be challenged to incorporate these new technologies as they emerge. In responding to this challenge, the following needs to be considered:
- New technologies are not necessarily better than existing technologies for teaching. They may however offer new opportunities for teaching differently and may enable new or better learning outcomes, as well as improving on existing learning outcomes.
- Old technologies rarely disappear completely as a result of popular new technologies. Older technologies become more focused and find a niche that they serve best.
- Most educators will be best served by not jumping on the latest technology bandwagon, but should wait a couple of years for a particular technology to reach at least the Gartner ‘slope of enlightenment’ before experimenting with the new technology.
- More important than the general characteristics of new technology is its design and application in education; in other words, how does it perform as an educational medium? Being a big success in the financial sector for instance does not mean technology will be automatically appropriate for education. Indeed, the technology may need to be heavily adapted or modified to be useful in the educational sector.
- Given the rate of change and the number of new technologies entering the market, educators need a strong framework or set of criteria for selecting and evaluating technologies, not just emerging technologies but also existing technology.
6. Activity (Reflective Thinking, Note Taking and Discussion)
This activity is optional. It is presented to facilitate your reflective thinking on the issues. There are no feedback. We encourage you to discuss these with your colleague.
Using Artificial Intelligence in education
- Do you agree with the three minimum requirements for modern AI: large data sets, powerful computing capacity, and powerful algorithms? Are there other possible applications of AI that do not need to meet these three criteria?
- Think of areas of teaching and learning that could generate large data sets even in a class of 30?
7. Key Takeaways
![]() |
Key takeaways from this lesson are:
|
---|